Mobility is one of the greatest challenges for smart cities. While technologies like the Internet of Things (IoT) have paved the way for intelligent transportation systems (ITS) that leverage data and sensors to create smart mobility solutions, gaps still remain. Leveraging the capabilities of IoT-enabled ITS with Virtual Reality (VR) and predictive analytics, a team in China combined the artificial and physical elements of a transportation system to develop a Parallel Transportation System (PTS) aimed at transforming how city planners approach urban mobility.
These IoT-driven systems have already made headway in many cities. They connect physical assets, such as connected vehicles, traffic systems, and infrastructure elements, and also connect social systems, such as economic development, emergency management, and urban planning.
Leveraging these deep sensing capabilities, the team’s PTS creates an artificial or virtual model of a city’s physical transportation system that runs and interacts parallel to the actual system. Instead of having the artificial model follow the physical system, like in most transportation simulations, the team’s approach instead opts for the physical transport system to live up to the artificial one. By conducting computational experiments, the team is able to predict changes in mobility and evaluate traffic control plans. Not only does this improve current transportation management, but it also expands the opportunities for building smarter and better connected cities.
“Parallel Transportation System methods might provide effective tools for the control and management of complex transportation systems that cannot be modeled precisely or experimented repeatedly,” said Fenghua Zhu, an associate professor at the State Key Laboratory for Management and Control of Complex Systems in China. “These methods are a natural extension of control systems and computer-based transportation modeling and simulations.”
Figure 1 outlines the architecture of the team’s parallel transportation system.
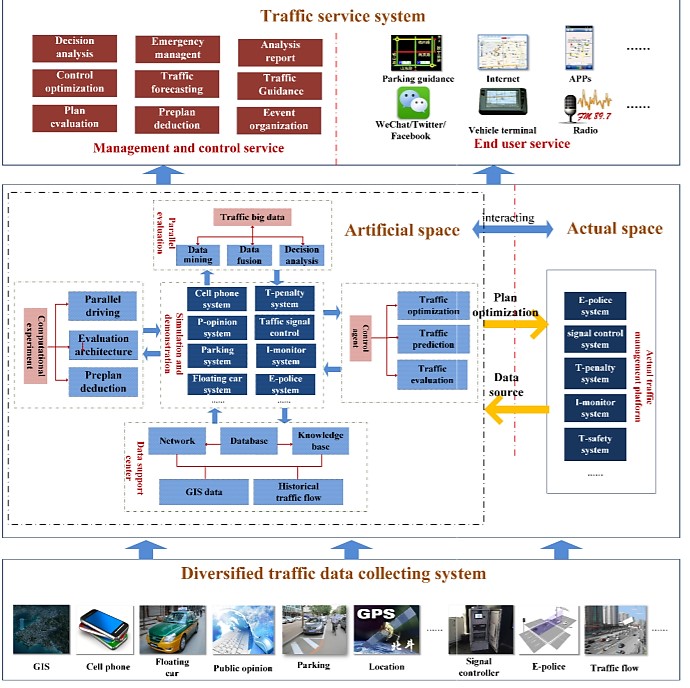
Figure 1: The architecture of the PTS based on IoT-enabled intelligent transportation systems
As with any ITS, IoT acts as the main driving force for seamlessly integrating the physical world with the virtual one. In the physical world, sensors around the city or embedded in mobile devices communicate with each other to form a distributed sensor network that collects regional traffic information, including traffic flow, occupancy, average speed, vehicle trajectory, and more. Data is also collected from social sensors, signals, and networking platforms such as Facebook and Twitter, where citizens share information in real-time. The data collected from the surrounding environments is then used to build an accurate representation in the virtual world using VR and artificial intelligence (AI).
Creating artificial traffic scenarios also allowed the team to generate and experiment with extensive virtual data, saving them time and minimizing costs when compared to the relatively “small” information collected from a city’s physical transport system.
“Similar to computer games, computational experiments can generate ‘artificial’ traffic behaviors and other related data using only population statistics and behavioral models,” said Zhu. “Computational experiments offer a platform or a ‘living traffic laboratory’ to analyze and synthesize transportation systems.”
The workflow is shown in Figure 2, where all physical experiments could be conducted and solved in the artificial world.
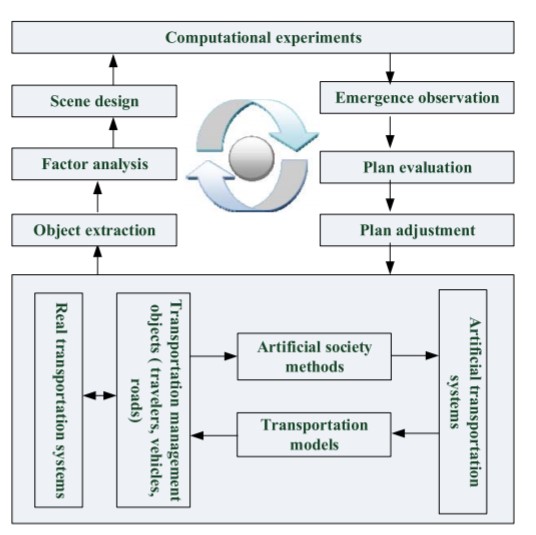
Figure 2: The workflow of the team’s PTS
To prove the effectiveness of Parallel Transportation Systems, field tests were carried out in more than 10 urban cities across China. These developing cities were undergoing changes in economic development, vehicle ownership, and traffic congestion. They also suffered from complex road networks and intersections that made traditional traffic solutions impractical to implement.
Figure 3 outlines the coverage and the architecture of the PTS in Binzhou, China. This is just one example of a city that benefited from the team’s PTS by improving traffic congestion, regulation, maintenance, and more.
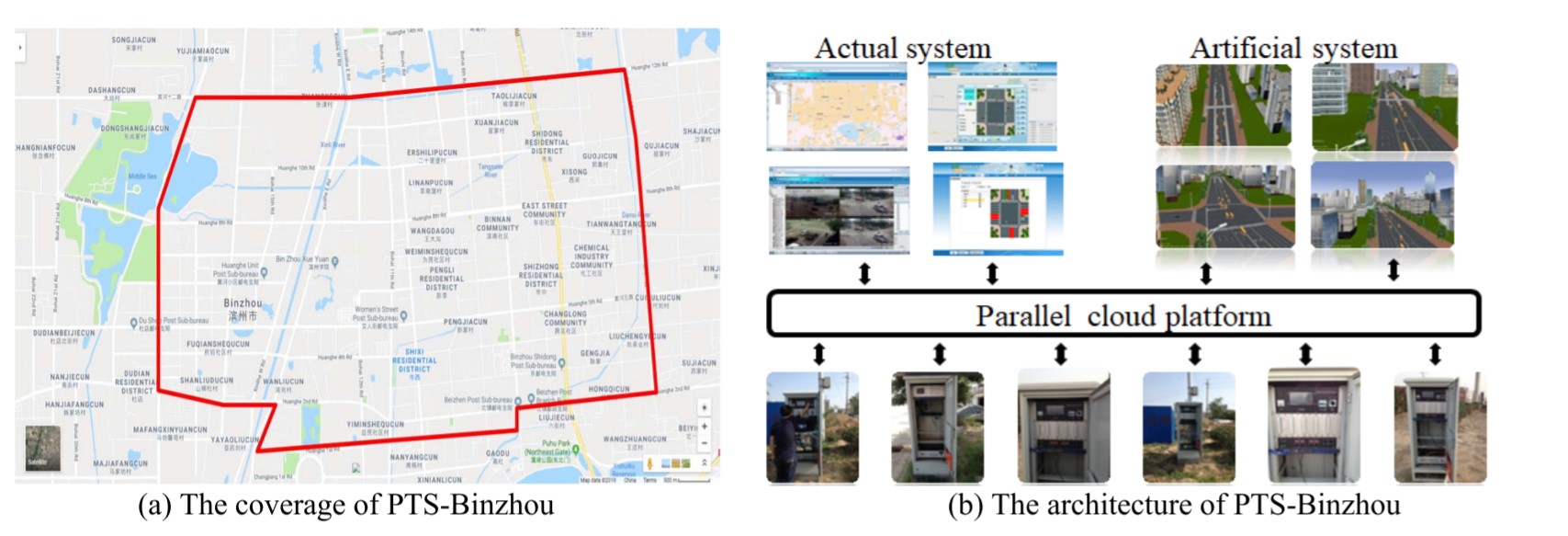
Figure 3: The PTS’ (a) coverage and (b) architecture in Binzhou, China
While Parallel Transportation Systems are not yet widely deployed, the team is already working on new upgrades as a next step. By further verifying the reliability of data collected from social sensors and enhancing the parallel learning AI algorithms, the team hopes to create a system that can make real-time decision making a reality in smart cities.
For more information on intelligent transportation or IoT, visit the IEEE Xplore digital library.