The holiday season is upon us, and that means big sales for retailers large and small. This year, many retailers are shifting focus from in-store to online promotions to reduce the spread of COVID-19. But as competition increases, retailers must ensure they don’t just draw shoppers online, but convert them into buyers. Using predictive analytics, researchers from Tsinghua University in Beijing, China, the Korea Advanced Institute for Science and Technology (KAIST) and Institute for Basic Science (IBS) in South Korea, believe they can help retailers identify their best customers seconds after shoppers get online, enabling improved targeting for promotions.
The team reviewed more than 31 million shopping logs captured during Singles’ Day – China’s top shopping day that takes place every November 11 – from large retailer Jingong Mall (JD.com). To understand the steps taken by potential buyers and classify them into categories, the data collected included consumers who purchased a product and those that didn’t, as well as those shopping online versus the retailer’s mobile app.
Researchers found purchasing behaviors tended to be different during Singles’ Day compared to a typical shopping day. Shoppers were more likely to make impulse decisions, and more first-time shoppers visited. They also discovered:
- About 18% of shoppers returned to the site multiple times, and these people were most likely to make a purchase.
- People who shopped via the store’s app made more purchases than those who shopped online. Just 39% of shoppers used the app, but they accounted for 56% of sales.
- Those who reviewed product details were more likely to make a purchase than those simply viewing discount information.
“One of the key findings was that predicting purchases is highly possible, even within a few seconds of observation,” said Muzhi Guan, a researcher for the Beijing National Research Center for Information Science and Technology at Tsinghua University in Beijing. “What is more interesting is the purchase intent rapidly increases within the first 30 seconds of browsing.”
Figure 1 illustrates the visiting patterns the retailer experienced over the course of the three days. For instance, varying levels of site visits could be attributed to specific promotions during the sales event. Some shoppers postponed a purchase until the last day, and simply browsed during the first two days.
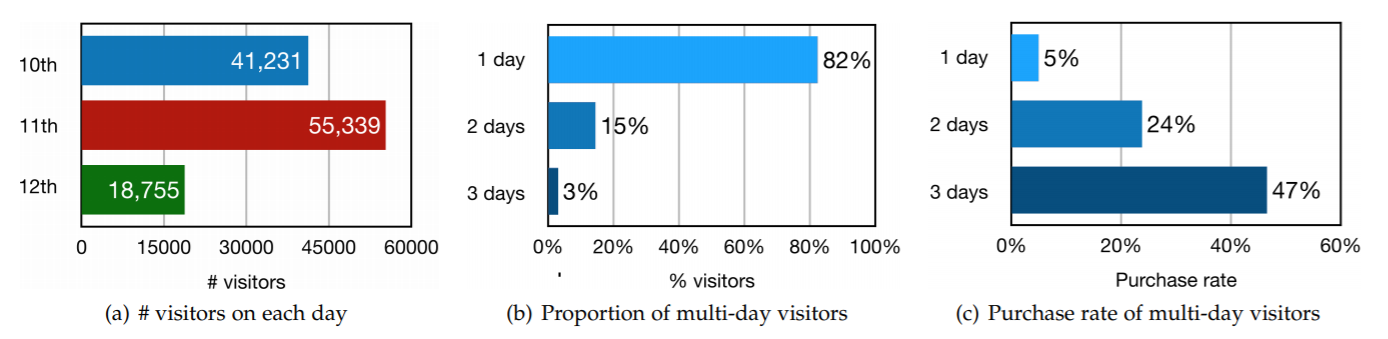
Figure 1: Key behaviors across the three days of Singles Day
Using these insights, the team was able to identify five categories of shoppers: browsing event and discounted pages (E); product listings from specific departments (L); product details (P); engaging in any kind of cart action, such as adding a product to a cart (C); and ordering an item (O). These action types are outlined in figure 2.
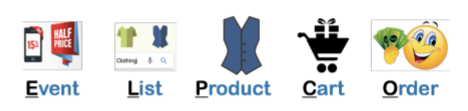
Figure 2: Five major shopping behaviors or actions
Actions E, L and P represent browsing behavior, while C and O represent purchasing decisions. By separating these two types of categories, the team was able to differentiate the state of consumers’ behaviors while shopping and their purchase intent once an item had been added to the cart – whether it’s a “save for later” item or a final purchase. Based on these patterns, the team was able to create an algorithm that took into account each step of the buying process, as shown in Figure 3.
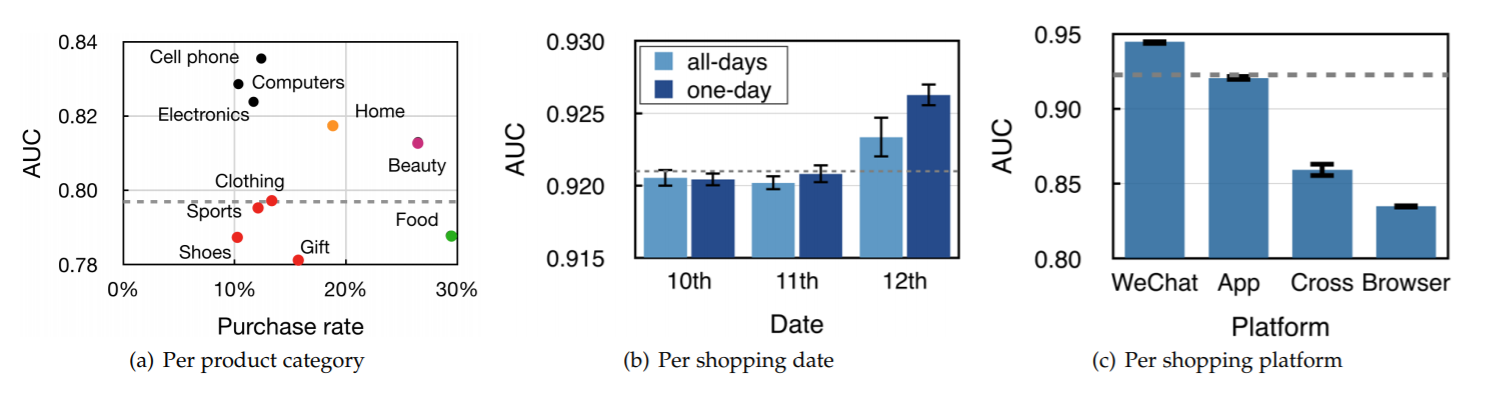
Figure 3: Purchase prediction by (a) category (b) date and (c) platform
A prediction model like this can help retailers improve the customer experience during large shopping events, better target promotions and discount codes, and allocate bandwidth to those most likely to buy when traffic is heavy – all within seconds of logging on.
According to Muzhi, with social distancing measures happening worldwide during this end-of-year shopping season, online shopping is expected to surge. Retailers will need to work harder than ever to win over shoppers, and those that use predictive analytics could have a more successful season.
For more information on predictive analytics and data mining, visit the IEEE Xplore digital library.