There are over 250 million people worldwide who have severe visual impairment, with 70 million entirely visually impaired (VI). VI individuals face several challenges when shopping, including: finding the right direction to desired commodities, distinguishing between similar-looking items, and not wanting to ask for help. More research is needed to provide user-friendly and practical assistance for VI individuals in complex environments, such as supermarkets.
An article in IEEE Transactions on Human-Machine Systems features research to improve the assistance experience for VI individuals during shopping. The researchers propose a fine-grained guidance strategy for a better shopping experience, including commodity selection, posture guidance, and occlusion inference. MobiEye, a user-friendly shopping assistance system for VI individuals, utilizes real-time video stream analysis technology. The experimental results show that MobiEye achieved a 13% improvement in pick-up success rate and a 12-second reduction in average pick-up time compared with other shopping assistance systems.
Understanding User Needs
The researchers interviewed VI participants to understand their shopping habits and challenges—and identified the core need for a shopping assistance system for VI shoppers. VI shopping behavior involves three phases: Getting to the supermarket, finding the shelf, and selecting the commodities. Access to supermarkets relies on mapping software and taxi apps, whereas finding the shelf relies on step counting or guidance. However, VI shoppers lack aids and guidance for selecting specific commodities.
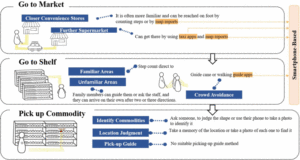
User journeys of shopping behavior.
After discovering that picking up the target commodity is the biggest shopping challenge for VI individuals, the researchers thoroughly analyzed this behavior and identified critical functions for an assistive system. For VI shoppers, picking up the target commodity is challenging as they rely on touch or assistance. The main issue is efficiently locating the target commodity at the front of the shelf. The solution focused on two critical aspects: commodity recognition and quickly guiding VI shoppers to the target. Considering participants' preference for wearable devices, mobile phones were chosen as our shopping assistance solution platform.
Introducing the MobiEye
The MobiEye shopping-assistance system aims to achieve two primary goals: identifying as many commodities as possible and providing direct guidance for quick item retrieval.
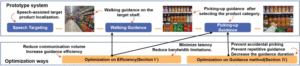
Flowchart of the shopping-assistance system for the VI shoppers.
The system consists of three modules: speech targeting, walking guidance, and picking-up guidance. The speech targeting module collects the VI's speech and identifies the desired item. The walking guidance module enables the VI to continuously track the item while walking towards it. The picking-up module provides detailed guidance by detecting the user's hand and the item's position.
The researchers invited VI participants to evaluate and optimize the prototype system as part of the testing. The initial evaluation of the system found suboptimal current deployment methods. The researchers proposed a mobile-edge co-computing strategy to improve the conflict between latency, accuracy, and communication volume. This strategy uses a motion predictor and communication gate on the mobile phone to filter the transmitted information, reducing the latency and communication overhead.
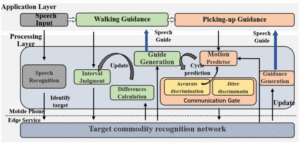
Architecture of edge-side collaborative computing system
Market Shopping Results
Following technical iterations, a field study in a supermarket environment was performed to assess the system's ease of use and practicality. Results show that MobiEye significantly improved the pick-up process for VI shoppers without significantly increasing guidance time.
In addition, MobiEye is a user-friendly guidance system for VI and has the best real-time prediction tracking.
The MobiEye optimization method outperformed the general guidance method, especially for smaller items. The system guides them efficiently with an average accuracy of 98.5%. We also improve the accuracy of picking up small commodities by 50% and reduce communication overhead by 75% compared with other shopping assistance systems. The researchers plan to enhance the system's recognition accuracy and capability to handle multi-person scenarios.
Interested in acquiring full-text access to this collection for your entire organization? Request a free demo and trial subscription for your organization.
Interested in expanding your knowledge on Human-Machine Interactions? IEEE offers continuing education with the Digital Transformation: Moving Toward a Digital Society Course Program to smartly implement digital tools into your organization.