How quickly a person blinks their eyes can indicate a number of different things—driver fatigue, deceptive behavior, and even neurological conditions like Parkinson’s disease. Technologies that can read a person’s behavior or intentions by spotting and analyzing facial features, such eye-blinking patterns, perform well under constrained indoor conditions, but not when the subject is in an uncontrolled environment. To address this, a group of researchers set out to develop a method to detect eyeblinks even in “wild” or unpredictable circumstances.
To take on the eyeblink challenge, an international joint team at the Huazhong University of Science and Technology in China, Southern Medical University in China, High Performance Computing, A*STAR in Singapore, and University at Buffalo, the State University of New York in USA started by building an eyeblink dataset, examining 20 popular movies such as The Matrix, A Clockwork Orange, and The Bourne Ultimatum, to capture samples that represent dramatic scene variations found in everyday life. This dataset (HUST-LEBW) includes variations of facial attributes, head poses, illumination, scene conditions, and imaging configurations that pose challenges to effective eyeblink detection.
As seen in Fig. 1 below, these movies mimic real-world visuals that can be processed for eyeblink detection.
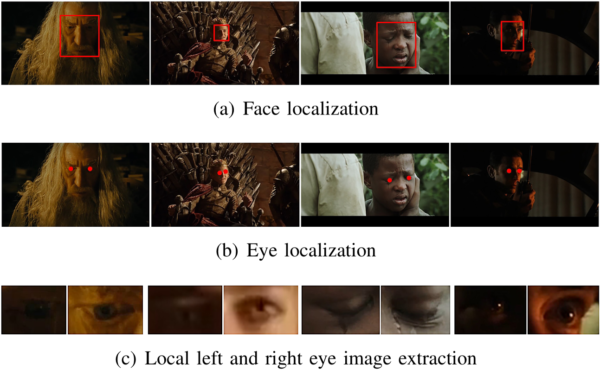
“Compared with randomly chosen volunteers, the professional actors in movies can perform eyeblink more naturally when they face the camera,” said Yang Xiao, an associate professor in artificial intelligence and automation. Using movies as the data source can help Xiao’s team extend the constructed dataset more easily in the future, at relatively low cost, he added.
They then developed a first-of-its-kind model of a spatial-temporal pattern recognition problem within eyeblink detection. The model uses a two-step verification program. First, the eye region is detected and an eyeblink is verified using a neural network to model the temporal sequence of a blink. Then, eye appearance and motion information are captured simultaneously to reveal the visual characteristics of the local eye region.
The researchers used the spatial-temporal sequence information to document the full movement of an eyeblink, from open to closed to open once more. They then used movies to test their eyeblink detection method, discovering that it worked better than other state-of-the-art eyeblink detection methods, especially regarding eye localization (locating the eye).
“In the future, we plan to apply more advanced techniques to enhance the performance, especially when a dramatic head pose or illumination variation happens,” says Professor Xiao.
As eyeblink technology advances, the team hopes it will be a helpful tool with a variety of applications, including reducing traffic fatalities, detecting concussions, increasing accuracy of field sobriety tests, monitoring for early signs of Alzheimer’s disease, and identifying fake videos online.
For more information on biometrics, visit the IEEE Xplore Digital Library.