Edge computing has emerged as a pivotal paradigm in the IoT and distributed computing era, bringing computation closer to data sources for improved latency and efficiency. However, the ubiquity of edge devices also exposes them to an increased risk of cyber threats, making cybersecurity a paramount concern.
At the International Conference on IoT in Social, Mobile, Analytics, and Cloud, held in October 2023, researchers explored the vital realm of cybersecurity fortification in edge computing environments, focusing on the synergy of deep learning, specifically Convolutional Neural Networks (CNNs), for intrusion detection and threat mitigation. The study investigated the fundamental concepts of edge computing, , highlighting its unique security challenges, including limited computational resources, diverse device types, and potentially untrusted network environments.
Edge Computing Challenges
With the proliferation of edge computing, the study addresses the challenges posed by resource constraints and real-time processing requirements.
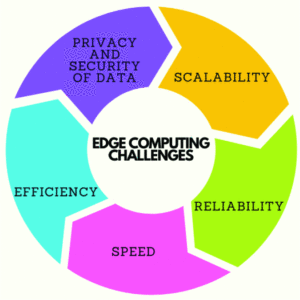
Edge Computing Challenge
To address these challenges, the researchers explored the concept of applying deep learning and CNNs in the case of intrusion detection and threat mitigation. In assessing the pacts between accuracy, resource utilization, and real-time responsiveness, critical factors for edge-based security solutions, the findings of this investigation not only shed light on the efficacy of CNNs in enhancing the cybersecurity of edge computing but also provide valuable insights into the practical implementation and optimization of deep learning models in resource-constrained environments.
As edge computing plays a pivotal role in various applications, from autonomous vehicles to smart cities, safeguarding these ecosystems against cyber threats becomes imperative. The insights and methodologies presented in this research contribute to advancing cybersecurity strategies in edge computing, paving the way for a more secure and resilient future in the age of distributed computing and the IoT.
Intrusion Detection in Edge Computing
With the proliferation of Internet of Things (IoT) devices and their integration into various sectors like healthcare, transportation, and manufacturing, edge computing has emerged as a paradigm that brings computational capabilities closer to the data source.
Edge computing environments often comprise many devices with limited resources such as computational power, memory, and energy. According to the researchers, innovative approaches are required to tailor intrusion detection mechanisms specifically for edge computing: a robust intrusion detection system should not only identify known attack patterns but also adaptively learn from new threats using machine learning techniques.
Deep learning emerges as a powerful approach for enhancing cybersecurity fortification in edge computing. It allows for developing sophisticated models capable of learning from massive amounts of data and making precise forecasts or classifications. While more conventional techniques can struggle to adapt to rapidly evolving cyber threats, CNNs excel at automatically extracting intricate patterns from complex datasets, enabling them to detect subtle anomalies that might indicate an ongoing attack or unauthorized access. Instead of merely reacting after an incident, this approach empowers organizations with early detection capabilities to prevent breaches before they cause substantial harm or disruption.
New Methods & Results
After discussing the literature review and previous work on this subject, the article shares new methods for securing edge devices and networks, encompassing lightweight encryption techniques, intrusion detection systems for edge devices, and secure data sharing between edge nodes.
Collectively, these methods strengthen the security posture of edge devices and networks, addressing the unique challenges posed by resource constraints and the dynamic nature of edge computing environments. The techniques provide varying levels of security and adaptability in different contexts, determined by network complexity, specific security needs, and the resources in each environment. The proposed CNN model achieved an impressive accuracy of 95.94%, signifying its ability to make accurate predictions.
Future Enhancements
As noted by the authors, there are several areas in which future enhancements can be made to further fortify edge computing through the synergy of deep learning, including:
- Advanced Adversarial Defense Mechanisms and exploring novel defense strategies like generative adversarial networks (GANs) or reinforcement learning algorithms will create IDSs with superior resilience against adversarial attacks.
- Real-Time Threat Intelligence Integration to ensure that edge computing systems possess an agile defense mechanism capable of detecting new attack vectors as they emerge.
- Explainable Deep Learning Models for Intrusion Detection would help security analysts understand and trust the decisions made by CNNs in real-world scenarios.
- Federated Learning for Distributed Intrusion Detection enables edge devices to collectively learn from diverse datasets without compromising individual user privacy, leading to more accurate and reliable intrusion detection systems.
These enhancements will improve the security capabilities and provide more efficient and effective threat detection and mitigation mechanisms. By understanding these state-of-the-art techniques, organizations can strengthen their ability to identify and mitigate digital threats at the edge, ensuring a robust and secure computing environment.
Interested in acquiring full-text access to this collection for your entire organization? Request a free demo and trial subscription for your organization.
Interested in expanding your knowledge on Cyber Security and Edge Computing? IEEE offers continuing education with these course programs to smartly implement digital tools into your organization:
- Hacking Your Company: Ethical Solutions to Defeat Cyber Attacks Course Program
- Cyber Security Tools for Today's Environment Course Program
- IEEE Introduction to Edge Computing
IEEE also offers the IEEE | IAPP Data Privacy Engineering Collection, delivering the most critical training, resources, and content for engineers and technology professionals tasked with understanding, maintaining, and protecting data privacy.