Construction robots and autonomous machines have gained considerable attention in recent years due to their potential to improve the productivity and quality of construction industry operations. According to researchers, a critical enabler of human-robot work collaboration at construction sites is a user-friendly interface to support the interaction between workers and robots. The proper communication interface can reduce workers’ mental stress and operational safety risks arising from the robots’ vague or incorrect understanding of instructions.
In a recent article published in IEEE Sensors Journal, researchers propose a system for recognizing construction workers’ hand gestures using wearable sensors on fingers. The system presented in the article is a wearable sensor-based system for automatically capturing and interpreting the hand gestures of construction workers. The procedure starts with synchronizing, normalizing, and smoothing finger motions. Then the motion data are extracted through a sliding window and fed into an enhanced fully convolutional neural network (FCN) for hand gesture recognition.
As noted by the authors, considerable research has been dedicated to the design of various types of human-robot interfaces based on visual displays, hand gestures, faces, speech, and natural language. Nonverbal communication, such as hand gestures, is deemed well-suited for noisy work environments and is commonly used on construction sites. They provide a standard method for workers to convey directions without complicated devices.
Automatic Gesture Recognition System Design
The proposed system’s design incorporates innovative methods to overcome the challenges often associated with construction sites. Specifically, several data preprocessing techniques are applied to reduce the data noise arising from irrelevant worker movements. According to the authors, the system comprises three modules. The raw signals from wearable sensors are fed into the data preprocessing module for synchronization, normalization, and smoothness. A sliding window method for processing all the incoming signals is designed to enable faster response. At the same time, the accelerometer and gyroscope data are fed into an enhanced fully convolutional neural network (FCN) to achieve gesture recognition.
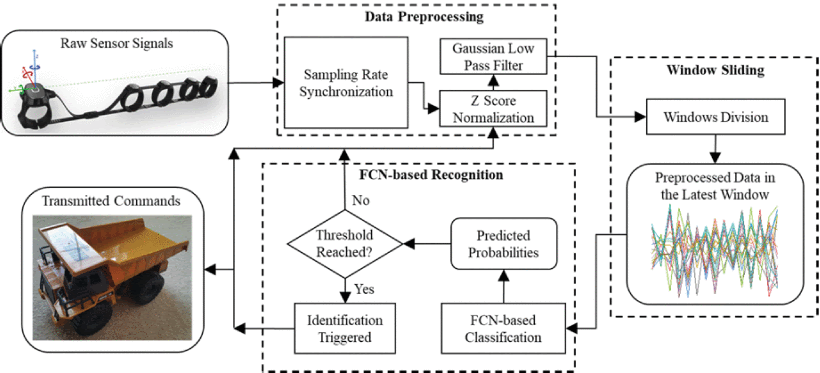
Overview of the proposed system.
Proving the System
A pilot study was conducted in a laboratory environment to test whether the proposed system could serve as an effective interface for workers to control and interact with robotic construction machines. The subject was asked to perform hand gestures captured by a Tap sensor connected to a computer. The sensory motion data obtained by the Tap sensor were input into the system and processed in real-time. Based on the recognition results, the corresponding instructions were sent to a remote controller, where the control signals were transmitted to operate the truck model.
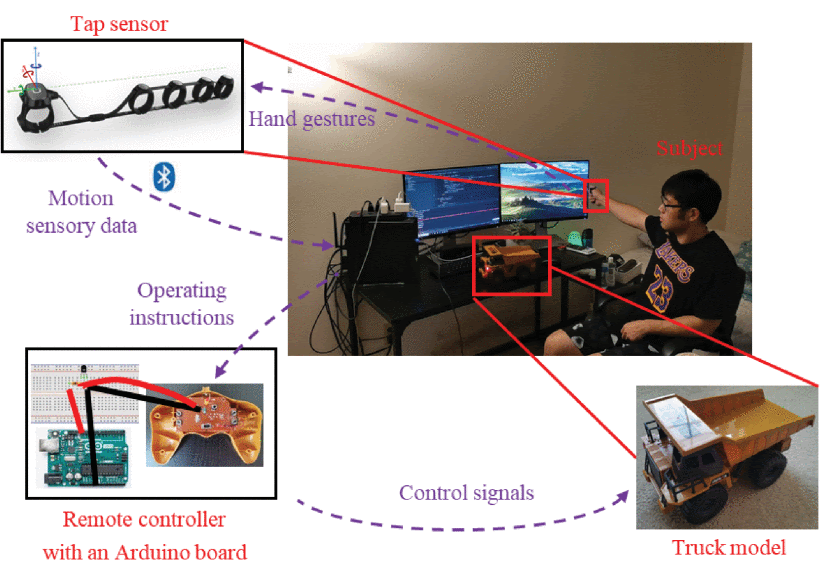
Pilot study setup and data flow.
The test results showed that the precision and recall achieved by the system were 85.7% and 93.8%, respectively. It was also successfully used to interact with a robotic dump truck. Compared with vision-based hand gesture classification, this study showed that sensor-based classification has the benefits of quicker response and strong anti-interference ability.
Future Research Directions
Although sensor-based classification has several benefits, as noted by the authors, the use of wearable sensors to capture and interpret the gestures of construction workers is still limited in practice. Vision-based methods only need cameras typically equipped on the intelligent construction robotic machine. Under this scenario, the machine can identify the worker through a detection and tracking module and thereby eliminating the cost of wearable sensors for the workers.
Construction robots can increase job site productivity and help overcome industry challenges such as labor shortages and safety risks. User-friendly interfaces are critical for advancing human-robot work collaboration and the growing use of construction robots. Future work should focus on including more classes of construction hand gestures into the dataset to train and test the sensor-based classifier developed in this study.
Interested in acquiring full-text access for your entire organization? Full articles available with purchase or subscription. Contact us to see if your organization qualifies for a free trial.