Similar to human drivers, adverse weather conditions in the form of heavy snow, fog and rain still pose as a major obstacle for autonomous vehicles. Like any new technology, companies have to address these challenges before self-driving cars can become mainstream. Using a deep learning (DL) framework along with sensors, researchers have developed a state-of-the-art vehicle detection and tracking system and dataset, aimed at solving how autonomous vehicles “see” their surroundings.
In order for self-driving applications to be effective in preventing accidents they need to accurately detect traffic obstacles in real-time. Typically, sensors such as cameras and light detectors are used to aid autonomous vehicles in tracking and monitoring. However, in the majority of cases, these sensors have trouble adapting in low-visibility situations, such as snow, rain and fog. As such, they are unable to read or track for road signs, pedestrians and other vehicles. While improving the image capturing capabilities in self-driving technologies has been a goal for many companies, tests for real-time detection under adverse weather conditions have been slow to rollout – until now.
The team’s proposed system uses automatic white balance (AWB) to improve the quality and contrast of the image captured. Specifically, it breaks down the illumination and reflection found in the image, while preserving the information needed to accurately detect objects such as, edge detailing, color naturalness and traffic scenes. The team’s restoration method is illustrated in Figure 1.
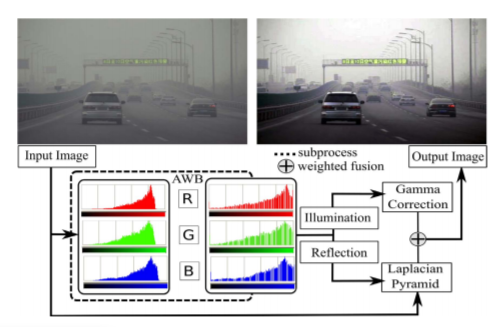
To keep track of vehicles in adverse weather, an online tracking system and algorithm was then introduced to address complications caused by missed detection, false positives and obstructions in the surrounding environment. The team also incorporated a new benchmark dataset, called Detection in Adverse Weather Nature (DAWN) to validate the effectiveness of their system.
DAWN is comprised of 1000 images that vary in terms of vehicle category, size, orientation and position. The images, which are taken from real-traffic environments, are divided into four primary categories: fog, rain, snow and sandstorms. Samples images from the DAWN dataset are shown in Figure 2.
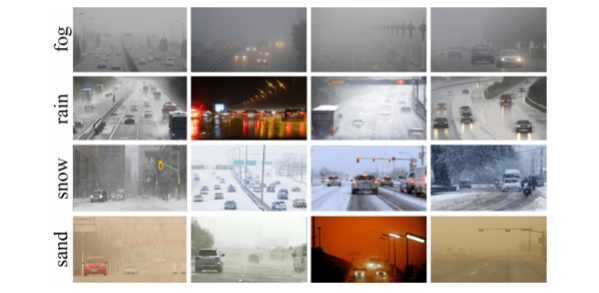
“DAWN is unique because it combines real-world images with different types of weather conditions and environments,” said Khan Muhammad, a researcher at Sejong University in Seoul. “Our system is unique due to its intelligent use of different technologies including DL for accurate vehicle detection and tracking.”
According to Khan, 21 state-of-the-art vehicle detectors were incorporated to compare and test the team’s system, which outperformed the others in its approach to accuracy and detection speed in various traffic environments. Overall, the unique characteristics of the DAWN dataset provides researchers with the ability to investigate aspects of vehicle detection that have not been previously explored.
Figure 3 outlines the team’s intended outcome of their vehicle detection and tracking system. Notably, not only can it be used in autonomous vehicles, but it can also be leveraged for surveillance purposes, especially in extreme weather conditions.
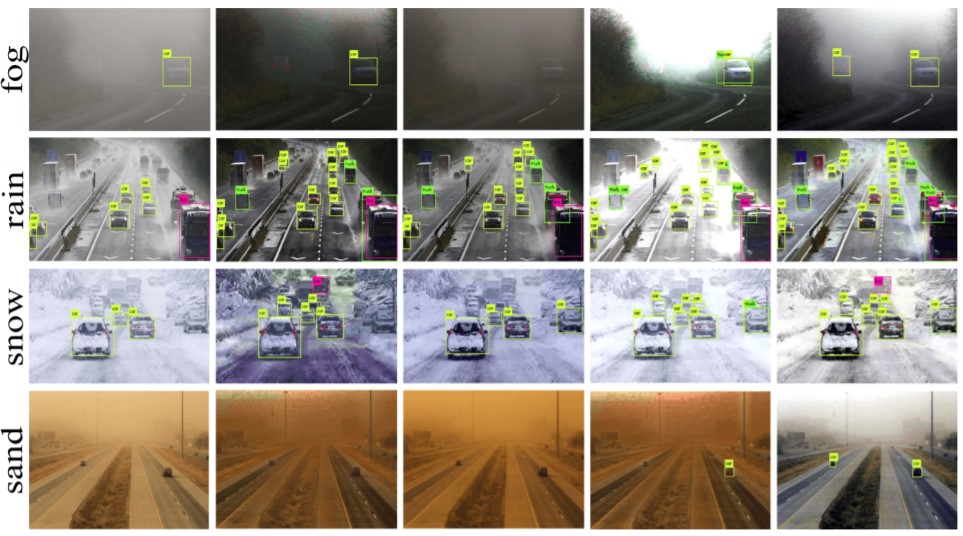
As a next step, Khan and his team are working on extending the DAWN dataset by adding in more data that covers natural disasters, such as hurricanes and severe thunderstorms – and they are not far off. DAWN has already shown to perform well in natural disasters that are similar to weather conditions already configured in the system.
From hype to reality, autonomous vehicles have had their fair share of speed bumps over the years, but with solutions like DAWN and AWB, companies are one-step closer in making them viable for everyday use.
For more information on deep learning and autonomous vehicles, visit the IEEE Xplore digital library.